Embedding Responsible AI Principles from Day One: An Ethics-by-Design Roadmap for AI Startups
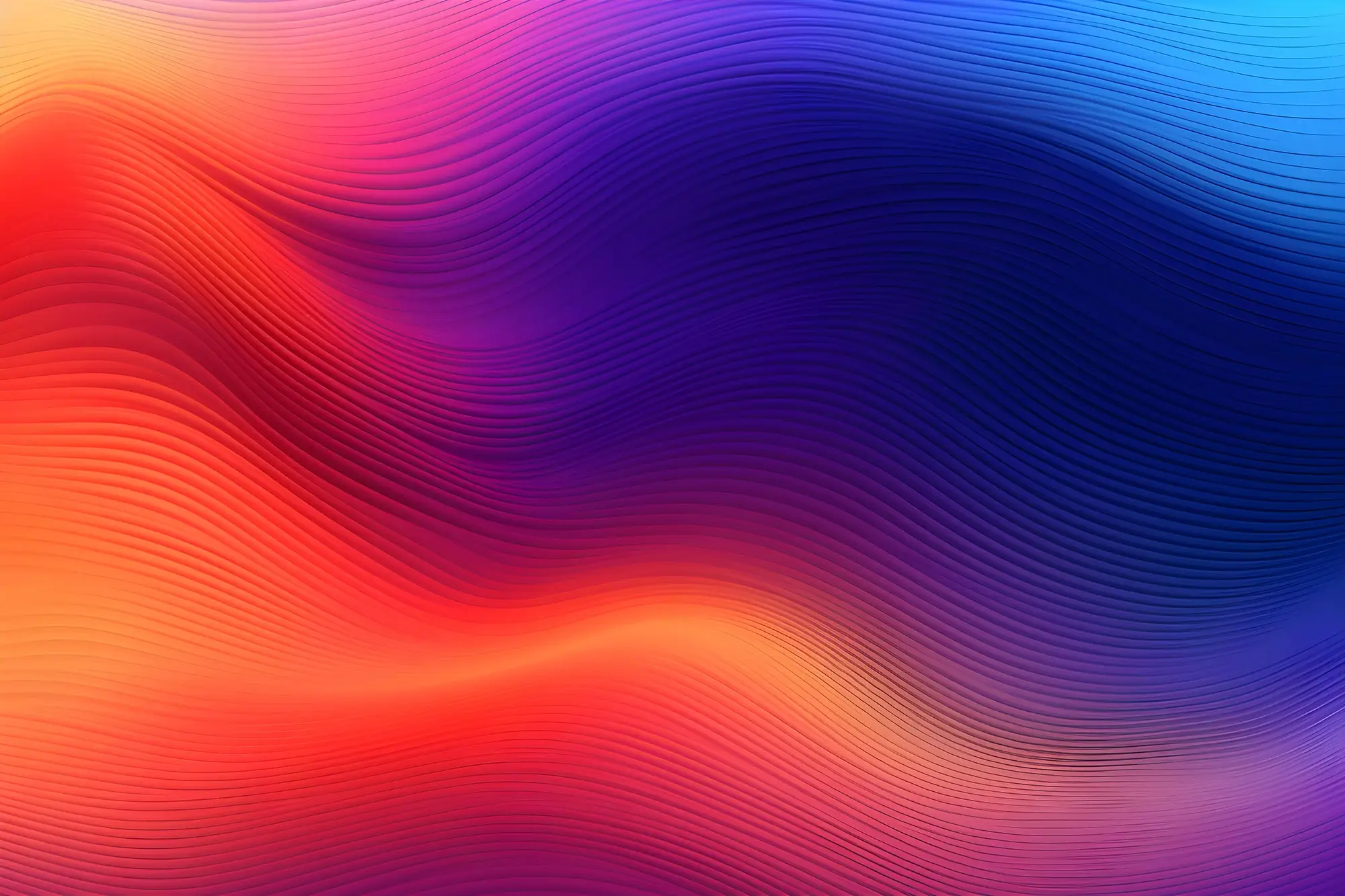
Embedding Responsible AI Principles from Day One: An Ethics-by-Design Roadmap for AI Startups
Introduction: AI startups have a unique opportunity to build trust and social value by integrating ethics into their products from the very start. Rather than treating ethical concerns as an afterthought or a compliance checkbox, an “ethics by design” approach means baking responsible AI principles into every stage of development – from data collection and model training to deployment and governance. This proactive strategy is not just morally sound; it also yields tangible benefits. Companies that invest early in responsible AI often accelerate innovation and gain a competitive edge. Conversely, failures to account for ethics can lead to serious pitfalls – for example, the COMPAS recidivism algorithm became notorious for racial bias in criminal justice recommendations, underscoring how unchecked AI can harm communities and reputations. This report provides a comprehensive guide for AI startup founders (and their investors) on implementing ethics by design. It covers what ethics by design entails, frameworks and tools for ethical AI development, strategies for bias mitigation, approaches to transparency, scalable governance structures, real-world implementation challenges, evolving regulatory expectations, and actionable steps (including a stage-by-stage roadmap) to build trustworthy AI products from inception.
Ethics by Design: Overview for AI Startups
Ethics by design is an approach to AI development that proactively embeds ethical considerations into the design and build process, rather than addressing ethics only after a product is built or when problems arise. The goal is to surface and handle potential ethical issues as early as possible in the innovation lifecycle. In practice, this means that from the moment a startup begins conceptualizing an AI-driven product, the team is also examining how that product could impact users, society, and stakeholders in both positive and negative ways. By integrating ethics at inception, startups can avoid the “band-aid” approach of patching issues later – for example, bolting on a privacy fix or bias mitigation after deployment – and instead prevent harm by design.
In the startup context, “ethics by design” translates to building on a foundation of ethical values from day one. This involves considering the impact on protected groups, user autonomy, and societal wellbeing from the start, as well as integrating principles like privacy, security, and fairness into the product requirements. For instance, a team following ethics by design might ask during initial design: Who could be adversely affected by our AI system? Have we minimized potential bias? How will we ensure user consent and data protection? Such questions guide the architecture and data choices early on. Ethics by design aligns closely with concepts like “privacy by design” and “security by design,” extending them to broader values.
It’s important to note that ethics by design is not a replacement for compliance or external oversight – it works in tandem with those efforts. The European Commission’s guidance on AI ethics emphasizes that adopting ethics by design does not preclude the need to meet all major AI ethics principles and legal requirements. In other words, a startup should embed ethical thinking into its product development and still conduct reviews, audits, and adhere to regulations. When done correctly, ethics by design can streamline later compliance: products built with fairness, transparency, and privacy in mind are naturally better aligned with emerging laws and customer expectations.
Finally, why should startups bother with ethics by design? Beyond avoiding ethical crises, it’s about trust and long-term success. Users and clients are more likely to trust AI systems whose developers clearly prioritized ethics from the outset. Public sentiment and investor trends show increasing scrutiny on AI misuse, bias, and safety. Starting with an ethical blueprint ensures that as the startup scales, it can proudly demonstrate responsible AI practices instead of retrofitting them under pressure. In sum, ethics by design for AI startups means making ethical and human-centric thinking an integral part of innovation – treating it as a core design criteria just like performance or user experience.
Frameworks and Methodologies for Integrating Ethics into AI Development
Implementing ethics by design can be greatly aided by established frameworks and methodologies. These provide structured processes and tools to help teams consider values and principles throughout AI development:
- Value Sensitive Design (VSD): VSD is a well-known methodology that integrates human values into technology design from the start. It employs an iterative approach of conceptual, empirical, and technical investigations to identify which values (e.g. fairness, privacy, autonomy) are relevant to the system, study how stakeholders perceive those values, and then design technical solutions that uphold them. In practice, an AI startup using VSD might begin by identifying key stakeholder groups (end-users, people impacted by the AI’s decisions, etc.) and their values, then brainstorm design features that honor those values. For example, if transparency is valued, the team might build an explanation interface into the product. VSD ensures ethical principles aren’t abstract ideals but are concretely addressed in design decisions.
- Human-Centered and Participatory Design: These approaches involve end-users and affected stakeholders directly in the design process. By co-designing with diverse users, startups can uncover ethical concerns that developers might miss. For instance, a participatory design workshop with representatives from a community that will use an AI tool can highlight cultural or social norms the AI should respect. This aligns with inclusive design, ensuring the AI system respects the context and needs of different user groups, thereby operationalizing principles like fairness and accessibility.
- Ethics Guidelines and Checklists: A number of high-level ethical AI frameworks have been published by governments and research bodies – such as the EU’s Ethics Guidelines for Trustworthy AI (which outline principles like human agency, technical robustness, privacy, transparency, diversity/non-discrimination, societal well-being, and accountability) or the OECD AI Principles. Startups can translate these into internal checklists or guiding questions during development. For example, the EU Trustworthy AI guidelines have been distilled into assessment lists; a startup might use these to self-evaluate their system at each milestone. However, many such guidelines are high-level and not immediately “actionable,” which has led to what some call the “principle–practice gap.” Teams often struggle to move from abstract principles to concrete implementation. To bridge this, resources like the Ethical OS toolkit and AI Ethics Canvas have emerged – offering scenario-based exercises and worksheets for anticipating potential ethical issues (like future misuse or unintended bias) and planning mitigations.
- Fairness & Accountability Frameworks: There are specific methodologies focusing on algorithmic fairness and accountability. For example, researchers have defined formal fairness criteria (e.g., demographic parity, equalized odds, predictive parity) and provided toolkits to evaluate these in models. Open-source libraries like IBM’s AI Fairness 360 (AIF360) and Microsoft Fairlearn offer dozens of metrics and algorithms to check for bias in datasets and models. A startup can integrate these tools into its model development pipeline – e.g., after training a model, run bias metrics to see if error rates differ across demographics, and if so, apply mitigation (we discuss mitigation strategies in the next section). Similarly, frameworks for accountability encourage documentation and audit trails. One example is the concept of “model cards,” a framework for transparent reporting of model details (originated by Google), which we’ll cover under transparency. Adopting such frameworks early helps operationalize fairness and accountability rather than leaving them as vague ideals.
- NIST AI Risk Management Framework (AI RMF): Published by the U.S. National Institute of Standards and Technology in 2023, the NIST AI RMF provides a comprehensive approach for organizations to integrate ethics and risk management into AI development. It outlines functions like Map (contextualize AI use and risks), Measure (assess metrics of trustworthiness such as fairness or robustness), Manage (mitigate risks), and Govern (establish organizational processes to oversee AI risk) as a continuous cycle. Startups can use this or similar frameworks to systematically identify ethical risks (e.g., bias, security vulnerabilities, lack of explainability) and address them with controls. The framework essentially prompts technical teams and leadership to think about ethical and societal impact at each step, turning broad principles (like “be fair”) into risk management actions (like “measure disparate impact on subgroups and reduce it”).
- Case Studies and Industry Guidelines: Learning from others’ experiences is also valuable. Large tech companies (Google, Microsoft, IBM, etc.) and industry consortia (Partnership on AI, IEEE) have published their responsible AI principles and sometimes their internal processes. For instance, Microsoft has released guidance on inclusive design for AI, and Google has shared lessons on implementing its AI Principles. While startups operate at a different scale, these can inspire methodology. IBM’s “Ethics by Design” mandate is one instructive example: IBM not only formed an AI ethics board but also trained all its 340,000 employees in an ethics-by-design methodology to ensure its principles translate into practice. A startup with 5 or 50 people can similarly ensure each team member is versed in the company’s ethical standards and knows how to apply them day-to-day (e.g., via regular team discussions on ethical cases or a short “red team” exercise to probe how their AI could be misused).
In summary, there is no one-size-fits-all methodology for ethical AI, but startups have a growing toolkit at their disposal. By leveraging frameworks like VSD for value-centric design, using checklists from trustworthy AI guidelines, and employing fairness and accountability tools, even a small company can systematically integrate ethics into its AI development lifecycle. The key is to move from high-level principles to concrete practices – making ethics an integral part of design reviews, testing protocols, and product requirements.
Bias Mitigation Strategies: Data Collection, Model Training, and Deployment
One of the most pressing ethical challenges in AI is bias – when AI systems systematically disadvantage certain groups or make unfair decisions due to skewed data or flawed algorithms. For AI startups, addressing bias from the start is critical. Bias can creep in at multiple stages: in the training data, in the learning algorithm, or even in how a model is used in the real world. An effective bias mitigation strategy is therefore multi-pronged, targeting data, models, and deployment practices.
- Mitigating Bias in Data Collection & Preparation: The old adage “garbage in, garbage out” holds true – if your training data is biased or unrepresentative, the AI’s outputs will be too. Startups should begin by sourcing diverse and representative data that reflects the populations on which the AI will act. This might mean deliberately collecting data from underrepresented groups or augmenting datasets to balance out skewed distributions. For example, if developing a hiring algorithm, ensure your training data isn’t predominantly from one gender or ethnicity. Techniques like re-sampling or re-weighting data can help here – e.g. oversampling minority class examples or assigning higher weights to underrepresented samples so the model learns their patterns. Additionally, data auditing is a crucial practice: analyzing the dataset for potential biases (such as whether certain categories are systematically underrepresented or whether labels themselves reflect subjective or biased judgments). Some biases are subtle – a photo dataset might have correlations like “kitchen” scenes mostly labeled with women. Recognizing these early allows the team to correct course (perhaps by gathering more images with diverse representation in kitchens, in this example).
- Bias Mitigation in Model Training (In-Processing): Even with the best data, models can inadvertently learn spurious or undesirable patterns. In-processing bias mitigation involves modifying the learning algorithm or objective to produce fairer outcomes. Researchers categorize bias mitigation methods into three buckets: pre-processing, in-processing, and post-processing. During training (in-processing), one common approach is to introduce fairness constraints or regularizers into the model’s loss function. For instance, a constraint might penalize the model if its error rate for one demographic is significantly higher than for another, thereby pushing the model toward more equal performance. Another technique is adversarial debiasing, where an adversarial network is trained simultaneously to try to predict protected attributes (like gender or race) from the model’s outputs; the main model is penalized if the adversary can successfully do so, effectively encouraging the model to not encode those biases. These advanced techniques may be heavy for very early-stage startups, but as the company’s capacity grows, integrating such algorithms can significantly reduce bias. Even without custom implementation, startups can use open libraries: for example, IBM’s AIF360 provides several in-processing algorithms (like Prejudice Remover, which adds a fairness term to the loss, or methods that enforce constraints like equalized odds during training). The key is that during model development, fairness should be treated as a metric to optimize, not just accuracy. By validating models on fairness metrics (e.g., checking if false positive rates are similar across groups) and tuning accordingly, startups can achieve more equitable models.
- Post-Processing and Deployment-Time Strategies: After a model is trained, there are still methods to mitigate bias in its outputs. Post-processing algorithms adjust the model’s decisions to improve fairness without retraining the model itself. For example, one might apply a threshold shift for different groups to equalize acceptance rates, or use a method like calibration to ensure probabilities mean the same thing for all groups. An illustrative technique is the “reject option” classifier: when the model is less confident (scores in a gray area), override or defer the decision to a human for certain sensitive cases – this can prevent automated unfair decisions. Importantly, in deployment, startups should also implement monitoring for bias. Real-world data often drifts from training data; continuous monitoring can catch if the model’s performance for a segment of users is degrading or becoming skewed. For instance, if a lending AI starts approving far fewer loans for a particular neighborhood over time, that warrants investigation. Regular bias audits – which some regulations now mandate annually for certain applications like hiring tools– can be part of the deployment plan. These audits might involve analyzing outcomes by demographic segments and verifying no new biases have emerged. Moreover, startups should enable user feedback mechanisms. If users can appeal or report an AI decision (say, a credit denial they believe is wrongful), that feedback loop can highlight bias that wasn’t apparent from internal tests.
- Bias Mitigation Culture: Beyond technical fixes, startups benefit from instilling a culture of bias awareness. Train your team on what bias in AI looks like and why it matters. Encourage developers and data scientists to always ask “Who might this model be unfair to?” and “How could this data be skewed?” as standard practice. Sometimes, a simple manual review of a sample of decisions with a diverse team can flag issues that metrics miss. Having team diversity helps too – a diverse team is more likely to catch biases and bring different perspectives on what “fair” means.
Real-world example highlights underscore the need for these strategies. When Amazon developed an AI resume screener, they found it was downgrading female applicants – reflecting past bias in hiring data. This issue was caught in testing, leading Amazon to discontinue that tool, but a startup could easily have deployed such a model unwittingly. By applying ethics by design and rigorous bias mitigation, startups can avoid launching products that bake in historical prejudices. Instead, they have a chance to build AI systems that actively promote fairness, or at least significantly reduce the inequities present in raw data. As one framework puts it, think of bias mitigation as achieving “algorithmic hygiene”: cleaning inputs, training with fairness in mind, and sanitizing outputs. A combination of these approaches – data diversification, fair algorithms, outcome monitoring – gives the best shot at an AI that is fair and trusted.
Approaches to Ensuring Transparency in AI Models and Decisions
Transparency is a cornerstone of responsible AI. It means users, developers, and other stakeholders can understand how and why an AI system makes its decisions. For startups, ensuring transparency can significantly boost trust – clients and consumers are more comfortable with AI when they can peek under the hood, even if just a little. Several practices and tools can help introduce transparency throughout model development and deployment:
Model cards have been likened to “nutrition labels” for AI models, offering a transparent overview of a model’s intended use, performance, and ethical considerations.
Documentation frameworks like Model Cards for models and Datasheets for Datasets are becoming industry standards for transparency. A Model Card is a short report accompanying a machine learning model that describes in plain language the model’s purpose, the data it was trained on, its accuracy across different groups, ethical considerations, and limitations or intended domains of use. For example, if a startup develops an AI for diagnosing skin conditions, its model card might note that the model was trained mostly on light-skinned individuals and thus has higher error rates on darker skin – warning users of that limitation. By openly acknowledging such details, the startup provides transparency and manages risk. Datasheets for Datasets perform a similar role for data, documenting how data was collected, what it contains (demographics, etc.), licensing, and any preprocessing. These practices force an internal review (developers must confront what’s in their data/model) and create external transparency (downstream users, regulators, or partners can review this documentation).
- Explainability Techniques: While documentation is high-level transparency, at the individual prediction level, explainability is key. Explainable AI (XAI) techniques aim to provide human-interpretable reasons for specific AI decisions. For startups deploying AI in high-stakes areas (like finance, healthcare, or hiring), offering an explanation for decisions isn’t just nice-to-have – it may be required by clients or laws. Techniques such as LIME (Local Interpretable Model-agnostic Explanations) or SHAP (SHapley Additive exPlanations) can be integrated to give users insight into why the AI reached a particular output. For instance, a fintech startup approving loans with AI could display a brief explanation: “Rejected because income is below $X threshold and credit history is short,” based on the model’s internal reasoning. These tools analyze the model’s behavior around the given input to identify influential factors. Another approach is using interpretable model designs (like decision trees or rule-based systems) for parts of the system, or visualizations that help make sense of complex models (for example, attention maps over an image to show what a vision model focused on). The goal is to crack open the “black box” enough that users are not left in the dark about AI-driven outcomes.
- Process Transparency: Startups should also consider transparency in their development process and governance. This means maintaining an AI audit trail – records of how models were built, what parameters and data were used, and what evaluations were done. If down the line an issue emerges, having this traceability is invaluable for accountability and fixing problems. Internally, teams can practice transparent decision-making: clearly documenting why certain design choices were made (e.g., why a less interpretable but more accurate model was chosen, and what mitigations were added to compensate). Some organizations even open up parts of their development to external review or “bug bounties” for ethics – inviting experts to critique their model for bias or security issues in exchange for reward, which promotes transparency and accountability. Startups may not have resources for formal bug bounties, but they can seek informal feedback from advisors or the community on their AI system’s design.
- User Communication: Transparency extends to how an AI startup communicates with users about AI use. At a minimum, being honest that a decision or service is AI-driven is crucial (for example, a chatbot should disclose it’s an AI, not a human, which aligns with proposed AI transparency regulations). Many AI ethics guidelines insist on the right to know when AI is involved. Furthermore, providing users with guides or FAQs about “How our AI works” can empower them. A startup might publish a simple whitepaper or blog for a general audience explaining what data the AI uses, how it makes decisions, and what safeguards are in place. Transparency in user experience can also be implemented: if appropriate, give users some control or insight, like a feature to see “Why did I get this recommendation?” on a platform, or the ability to correct the AI (thumbs up/down, feedback forms).
- Calibration of Transparency: One challenge is finding the right level of transparency. Too much detail can overwhelm or reveal sensitive IP, while too little breeds mistrust. Startups should calibrate transparency to the audience. Investors or enterprise clients might appreciate a more technical due diligence report, whereas end consumers benefit from plain-language summaries or visual explanations. The guiding principle is clarity: offer clear, truthful, and relevant information without expecting the user to have an AI PhD to grasp it. Over time, external expectations are rising – for instance, the EU AI Act will likely require certain AI systems to provide information on how they work and their limitations. Getting ahead by implementing transparency practices now will make it easier to comply with such rules (discussed further in the regulations section).
In summary, ensuring transparency means making the opaque workings of AI more visible and understandable. Startups can employ documentation like model cards to be upfront about their models’ scope and limits, use explainability techniques for decision-level insight, and communicate openly about their AI use and development process. By doing so, they build credibility and trust, showing users and regulators alike that they have nothing to hide and are confident in the responsibility of their AI.
Scalable Governance Structures and Policies for Responsible AI
As an AI startup grows from a scrappy team into a larger organization, informal practices need to evolve into formal governance. Responsible AI governance refers to the internal structures, roles, and policies that ensure ethical principles are consistently applied and maintained over time. Startups should design governance that can scale – what works when 5 people are building one AI product should adapt when 500 people are deploying multiple AI systems. Here are key elements of scalable AI governance:
- Foundational Ethical Principles and Policies: Early on, founders should articulate a set of AI ethics principles or a company AI manifesto (e.g. “Our AI will be fair, transparent, secure, and human-centered”). This sets the tone and gives a reference point for decision-making. As the startup grows, these principles should be translated into written policies and standards. For instance, a Responsible AI Policy might state that all AI products undergo a fairness check before release, or that user data will not be used without consent. Policies might cover areas like data governance (how data is collected, annotated, and stored ethically), model development (requirements for testing, documentation), and deployment (e.g., human oversight for certain decisions, incident response if something goes wrong). What’s important is to get these principles out of founders’ heads and into a form that can be communicated and trained upon. Over time, these policies should be revisited to stay up-to-date with new ethical insights and laws.
- Roles and Responsibilities: In a tiny startup, one person can wear the “ethical risk guardian” hat. Typically, this might be the CTO or a co-founder ensuring the team discusses and addresses ethical issues. However, as employee count increases and projects multiply, it’s wise to designate specific roles or teams for AI ethics and compliance. Some startups form an internal ethics committee or working group once they hit a certain size (perhaps when moving from prototype to product, or when planning to deploy a high-impact AI). This group can be cross-functional – e.g., include a technical lead, a product manager, maybe someone from legal or operations, and even an external advisor periodically. Their mandate is to review AI initiatives for alignment with ethical principles and to handle any dilemmas that arise (like if the growth team wants to use personal data in a new way, the ethics group weighs in on propriety). Eventually, companies may appoint a Chief AI Ethics Officer or Responsible AI Lead. For example, some companies have created roles like “AI Ethics Program Manager” or expanded the remit of a Chief Privacy Officer to cover AI ethics. The IBM case study shows a mature model: IBM convened an internal AI Ethics Board to govern AI strategy, but also empowered a network of “focal point” employees in each business unit to implement ethics-by-design day-to-day. A startup won’t have that scale, but the lesson is to imbue ethics responsibility throughout the org chart – not just at the top.
- Ethics Training and Culture: Governance is not just structure, but also culture. As new employees join, especially those building or selling the AI product, they should be onboarded not just on the tech stack and business model, but also the company’s ethical standards. Regular training sessions or workshops on AI ethics can keep awareness high. These don’t need to be dry lectures – they can be interactive, like scenario discussions (“What would we do if our health diagnostic AI misdiagnoses a patient? How do we design to prevent harm?”). Encouraging an open culture where anyone (junior engineer or senior exec) feels comfortable raising a concern about the AI is vital. Whistleblower or feedback channels for ethical issues (even informal, like an email alias or part of retrospectives) ensure governance isn’t just top-down but bottom-up. Essentially, every employee becomes part of the governance fabric when empowered to uphold ethical practices.
- Stage Gates and Review Processes: A practical way to enforce ethics as the startup grows is to integrate it into existing workflows. For example, instituting an “AI ethics review” checkpoint before a product launch or major update. This could be a meeting or document where the team must demonstrate what they have done about bias, privacy, security, etc., and an approver (could be the ethics committee or an exec) signs off. Similarly, code reviews might include items related to ethical considerations (“Did we remove personal identifiers not needed for the model?”). Some organizations create risk assessment templates – essentially a questionnaire for the project manager to fill out describing potential ethical risks and mitigations for their AI project. By formalizing these reviews, ethical compliance becomes as standard as, say, quality assurance or security testing in the development pipeline.
- External Advisory and Accountability: As a startup matures (especially if it deals with sensitive AI applications), it can be valuable to seek external perspectives. Some startups form Advisory Boards that include ethicists, legal experts, or representatives of impacted communities to periodically review and advise on the company’s AI use. This adds credibility and catches blind spots internal people might have. In addition, being transparent with investors and boards of directors about AI risks is key to scalable governance. Company leadership should brief the board on AI ethics efforts and risks – a trend that’s increasing as corporate boards realize AI governance is a part of their oversight duties. Setting this precedent early will make governance a shared responsibility at the highest level. Furthermore, as the company grows, engaging with industry consortia or standards bodies (like the Partnership on AI, or ISO AI standards committees) can help stay ahead of best practices and signal the startup’s commitment to responsible AI.
- Continuous Improvement: Scalable governance is not “set and forget.” There should be mechanisms to update policies and practices as new challenges emerge. For instance, if a new type of bias is discovered in the product, feed that back into the policy (maybe now require bias checks for that attribute going forward). If regulations change, governance processes need updating (more on this next). Periodic audits of the governance system itself can be useful: maybe once a year, reflect – are our ethical guidelines still relevant? Did any incidents slip through? Are employees following the procedures or are they too cumbersome? A lean startup can pivot not only its product but also its governance as needed.
In essence, scalable AI governance starts with strong leadership commitment and simple practices, and evolves into a more formal system of roles, committees, and policies as the company expands. By the time a startup becomes a scale-up, it should have in place an organizational structure that systematically manages AI risks and ethics – much like how companies have structures for financial governance or cybersecurity. This ensures that growth in size or complexity doesn’t dilute the initial ethical intentions. Instead, those intentions are codified and reinforced through governance, keeping the company on a path of responsible innovation.
Real-World Challenges in Implementing Responsible AI
While the case for responsible AI is strong, AI startups face very real challenges in trying to implement these practices. It’s important to acknowledge and plan for these hurdles:
- Resource Constraints: Early-stage startups often operate under tight budgets and timelines. Conducting extensive ethical impact assessments or bias mitigation can seem like a luxury when the team is racing to get an MVP out. Indeed, implementing responsible AI measures can require additional resources and capabilities, and some fear it could slow down product development. Responsible AI projects are sometimes more complicated and costly to scale compared to those solely optimizing for performance. For example, collecting a diverse dataset might take extra time and money compared to using an available but homogeneous dataset; similarly, building an explainability interface might add development overhead. Startups may worry about this trade-off between ethics and speed. The challenge is real, but it can be managed by prioritizing high-impact, low-effort ethical interventions first, and communicating to investors why certain ethical investments are necessary to avoid bigger costs later (like a PR disaster or product recall due to an undetected bias issue).
- Lack of Expertise: AI ethics is interdisciplinary, and a small startup team may not have an in-house ethicist or a diversity of perspectives. Engineers and data scientists might be experts in coding and math, but less familiar with social science research or legal norms around discrimination, for instance. This gap can make it hard to foresee issues. A team might not realize a seemingly innocuous feature has ethical implications because no one in the room has the lived experience or training to spot it. Furthermore, the field of AI ethics is evolving; keeping up with best practices (what fairness metric to use? how to explain an AI decision effectively?) is a challenge when the team is heads-down building technology. Many startups address this by seeking external mentors or advisors in responsible AI, or leveraging community resources (there are now open communities and forums on AI ethics where practitioners share knowledge). Over time, as the startup grows, hiring someone with expertise in responsible tech or training existing staff can fill this gap.
- High-Level Guidance vs. Concrete Implementation: As noted, there’s been an explosion of AI ethics principles published by governments, academia, etc., but translating them into action isn’t straightforward. Startups can be unsure how to operationalize concepts like “ensure AI respects human dignity” in their specific product. The lack of standardized tools or certified “ethical AI” processes means teams often navigate in a gray area, figuring it out as they go. This can lead to analysis paralysis (not knowing what’s enough) or, conversely, ethical oversights (thinking something is fine because no guideline said otherwise). It’s a challenge of turning theory into practice. Using concrete frameworks like those in this report (checklists, bias testing toolkits, etc.) can help, but it requires deliberate effort to adapt general ideas to one’s context.
- Cultural and Market Pressure: Startups face intense pressure to grow, deliver features, and beat competitors. This “move fast and break things” culture that’s often celebrated in tech can conflict with the careful deliberation that responsible AI sometimes needs. Founders might fear that spending extra weeks on an ethics review could mean losing the first-mover advantage. Additionally, investors might push for rapid scaling, and unless they are aligned on ethics (which, encouragingly, many are increasingly aware of), they might not prioritize funding “non-functional” work like ethics infrastructure. This cultural challenge requires strong conviction from the leadership that doing the right thing is ultimately good for business – and being able to persuasively articulate that to the team and investors.
- Ethical Uncertainty and Trade-offs: Ethical dilemmas often involve gray areas and trade-offs with no easy answers. A classic example is the trade-off between model accuracy and fairness – sometimes improving fairness might decrease accuracy on the current data. A startup building a medical AI may face a dilemma: should it optimize for overall accuracy, or ensure the model is equally accurate across all demographic groups even if it means a slightly lower average accuracy? These decisions can be tough, and there might not be a consensus even within the team. Similarly, making an AI more explainable might make it less complex and potentially less accurate. Deciding the right balance requires thoughtful discussion and sometimes consultation with external stakeholders or ethicists. Startups must navigate these choices often without clear guidance or precedent, which can be stressful and risky.
- Maintaining Ethics Under Scaling and Pivoting: As a startup’s product scales or the business model pivots, new ethical challenges can emerge. For instance, a startup that initially offered a biased AI tool to a small pilot group can fix issues relatively quietly. But if they scale to millions of users and then a bias is found, the impact is widespread and the remediation is much harder (both technically and in terms of public relations). The process of scaling “can introduce obstacles and complications to realizing or preserving responsible AI adherence”. Technical infrastructure might strain under adding fairness checks, or new contexts might break assumptions (an AI deployed in a new country could run afoul of local cultural norms or laws). Ensuring that responsible AI practices themselves scale – that audits, monitoring, stakeholder engagement, etc., keep up with the size and scope of deployment – is challenging. Pivots are another issue: a startup might repurpose its AI for a different use-case (say, an algorithm meant for diagnosing skin rashes is now used for diagnosing all sorts of diseases). With new use, new risks: the skin AI might not work well on other illnesses or could present new biases. The team must quickly identify these and adapt their ethical safeguards, essentially re-opening the design questions under new conditions.
- External Environment Challenges: Sometimes the environment a startup operates in makes responsible AI harder. For example, if all available datasets in a field are biased, the startup might not have the means to create a less biased dataset from scratch. Or regulations might be unclear, leaving startups uncertain about how far to go or what standard they’ll be held to. Also, users themselves might misuse the AI in unforeseen ways (like using a general language model to generate hateful content), which the startup then has to address – essentially dealing with ethical issues created by users’ actions. These external factors require adaptability.
Despite these challenges, many startups find that being aware of them is half the battle. By anticipating where they might struggle, founders can proactively seek solutions – whether that’s allocating a bit of budget for an ethics consultant, scheduling an “ethics sprint” to focus on these issues, or choosing investors who explicitly support building responsible technology. Moreover, regulators and larger companies are starting to provide more guidance and tools, which will gradually ease the burden on startups. The road to responsible AI can be bumpy, but the long-term rewards (avoiding costly failures, building brand trust, aligning with upcoming laws) strongly justify the effort.
Evolving Regulatory Expectations and Staying Compliant
The regulatory landscape for AI is rapidly evolving, as governments worldwide grapple with how to ensure AI systems are safe, fair, and transparent. AI startups need to keep a finger on the pulse of these developments, because compliance (or readiness to comply) will increasingly be a differentiator and requirement for doing business. Here’s an overview of key regulatory expectations around AI ethics and how startups can stay compliant:
- The EU AI Act: In 2024, the European Union finalized the AI Act, the first comprehensive legal framework for AI. Think of it as akin to Europe’s GDPR, but for artificial intelligence. The AI Act uses a risk-based approach: it categorizes AI systems into risk levels (unacceptable risk, high risk, limited risk, minimal risk). Unacceptable-risk AIs (like social scoring systems or certain types of real-time biometric identification in public) will be outright banned. High-risk AI systems (which likely include things like AI for hiring, credit scoring, medical devices, etc.) will be allowed but under strict requirements. These requirements for high-risk AI likely include: rigorous risk assessments, high-quality training data to minimize bias, transparency to users (e.g. people must be notified when interacting with AI in certain contexts), human oversight, robustness and cybersecurity, and documentation for compliance (the provider must maintain technical documentation and logs to prove compliance). The Act also sets penalties for violations – with fines up to €30 million or 6% of global turnover for the most serious offenses, which is on par with or even higher than GDPR fines.
How startups can comply: First, determine if your AI system would be considered high-risk under the EU AI Act. The Act provides use-case categories (like employment, education, essential private/public services, law enforcement uses, etc.). If you’re in one of these, you’ll need to prepare for compliance even if you’re not in the EU – the law has extraterritorial effect (if your product affects people in the EU, it applies). Startups should start aligning with the Act’s provisions before it fully comes into effect (there is likely a grace period of a couple years for enforcement). This means implementing a quality management system for AI, doing thorough testing for bias and robustness, keeping documentation (like those model cards and data sheets, which will help in creating the technical file for regulators), and possibly engaging external auditors or conformity assessment bodies if required. There are tools and checklists emerging (for example, an AI Act compliance checker for SMEs is available to help startups self-assess). Being proactive here is key – startups who can say “we meet the EU AI Act requirements” will have an easier time entering EU markets and gaining enterprise customer trust.
- United States and Other Regions: The U.S. as of 2025 does not have a single federal AI law like the EU’s Act. However, there’s a patchwork of regulations and a strong signal that more is coming. For instance, many states have been introducing AI bills – in 2024, bills were introduced in at least 45 U.S. states, with 31 states enacting some form of AI-related laws (though many of these are establishing task forces or focusing on specific domains). On the federal level, there are ongoing discussions and proposals – like the Algorithmic Accountability Act (reintroduced in Congress) which would require impact assessments for AI systems used in critical decisions, or the “AI Bill of Rights” blueprint introduced by the White House (which is not law, but a set of principles to guide AI development, emphasizing protection from unsafe or discriminatory AI, data privacy, transparency, etc.). Regulatory agencies are also stepping in using existing laws: the FTC in the US has warned that biased AI outcomes could be prosecuted under anti-discrimination law or consumer protection law (misleading claims about AI or negligent data practices).
How to comply/stay prepared: Startups targeting the US market should follow guidance from bodies like NIST (the AI Risk Management Framework mentioned earlier has official backing) and sectoral regulators. If you’re in healthcare, look at FDA’s emerging framework for AI/ML-based medical devices. If in finance, look at guidance from CFPB or Federal Reserve on algorithmic credit decisions. A very practical step is implementing AI impact assessments voluntarily – essentially a self-check document analyzing how your AI might impact fairness, privacy, etc., and how you mitigate it. This mirrors what proposed laws would require and puts you ahead of the curve. Additionally, adhere to privacy laws like GDPR (in Europe) and CCPA/CPRA (in California) when handling personal data for AI – these laws already impact AI via data restrictions. Ensure you have user consent for data usage, and provide opt-outs for automated decision-making where required.
We already see specific local laws: New York City’s Local Law 144 (effective 2023) requires that automated hiring tools be audited for bias annually and that results be made public. Startups providing AI HR solutions must comply in that jurisdiction, which likely foreshadows broader requirements in the employment domain. Worldwide, other countries like Canada, the UK, and Australia are also formulating or updating AI guidance (the UK, for example, is taking a principles-based regulatory approach, asking existing regulators to incorporate AI considerations under principles like safety, transparency, fairness).
- Transparency and Explainability Requirements: Regulatory expectations consistently emphasize transparency. The OECD AI Principles (which many countries including the US and EU have adopted) highlight transparency and explainability as a key tenet. The EU AI Act will require that users are informed when they are interacting with an AI (e.g., deepfake or bot disclosure) and that explanations be available for certain high-risk AI decisions. Financial regulations often require an explanation for decisions like loan rejections (even if made by AI). Startups should thus build in the capability to explain and document decisions not just for users, but for regulators if asked. An example from the EU’s GDPR: if an automated decision has legal or similar significant effects on someone, they have the right to request human review – which means you need to have enough transparency internally to facilitate that review (or maybe avoid fully automated decisions in those cases).
- Robustness and Safety Standards: Another theme in regulation is requiring AI to be tested for robustness (resistance to manipulation or adversarial inputs) and accuracy. If you are deploying, say, an AI chatbot, how do you ensure it won’t produce disinformation or harmful content? Regulators are concerned about safety (e.g., an AI in a car or a medical device must not malfunction and harm people). The forthcoming standards like ISO/IEC 42001 (an AI management system standard, similar to ISO 9001 quality management but for AI) will likely incorporate these aspects. Startups aiming to be compliant should adopt good software engineering and QA practices: stress testing AI models, validating on various scenarios, and controlling quality of data. They should also maintain logs so that if something goes wrong, it can be audited (some laws might require keeping records of AI system decisions for a period).
- Governance and Accountability: Regulations won’t just look at the AI model in isolation; they will expect organizations to have governance in place. The EU AI Act, for example, effectively forces providers of high-risk AI to have risk management systems, data governance practices, and post-market monitoring. This aligns with what we discussed in the governance section – having those structures will not only help ethically but also legally. Additionally, liability regimes are evolving. If an AI causes harm (like a faulty decision), who is accountable? Startups should track regulatory discussions on AI liability. Ensuring a human-in-the-loop or at least human-on-the-loop oversight for critical decisions can both improve ethics and reduce legal risk (since you can argue a human had final say if something went awry).
Staying compliant in this fluid landscape means being proactive and informed. Some tips for startups:
- Assign someone to monitor AI policy news. This could be an interested team member or an external legal advisor who gives you briefings. The EU AI Act, for example, will have specific guidance published for compliance – know when those come out.
- Engage with compliance tools: As mentioned, there are checklists and frameworks (NIST, ISO draft standards, etc.). Even if voluntary now, they often foreshadow mandatory requirements.
- Document everything: If you do your due diligence (bias testing, stakeholder consultation, etc.), document it. This becomes evidence of compliance or at least of good-faith effort, which can be invaluable if a regulator knocks on your door or if you need to certify your product.
- Consider certifications: In the near future, we may see certifications or seals for ethical AI (some initiatives already exist, like certification for privacy compliance or ISO standards adoption). Achieving a certification can simplify demonstrating compliance to clients and regulators.
- Legal counsel: As the startup grows, consult with tech policy lawyers to review your AI use. Early advice can clarify which laws (current or upcoming) apply to you. For example, a lawyer might point out that your healthcare AI will need CE marking in Europe as a medical device (which has its own set of regulations for safety and efficacy).
Regulation need not be viewed as a barrier; often, it codifies what responsible startups are doing anyway. By aligning your practices with ethical best practices, you’re likely aligning with the spirit of these laws. Indeed, many leaders acknowledge that mitigating AI risks (the goal of most regulations) is not just compliance drudgery but can be a competitive advantage and a foundation for long-term success. Startups that internalize this and prepare early will navigate the evolving regulatory maze more smoothly than those who delay action until the rules hit hard.
Key Steps and Actionable Guidance for Building Trustworthy AI Products
Finally, what are the practical steps startup founders (and investors) can take to ensure they build and fund trustworthy, responsible AI? Below is a distilled guide:
- Define and Embrace Ethical AI Principles: Clearly articulate a set of guiding principles for your AI (e.g., “We will avoid biased outcomes,” “We prioritize user privacy and safety,” “We value transparency.”). Get buy-in on these at the leadership level and communicate them to the whole team. This forms a compass for decision-making. Investors should inquire about a startup’s AI ethics principles during due diligence – it signals that this matters for backing a company.
- Embed Ethics into Design and Development: Don’t treat ethics as a one-off review at the end. Incorporate ethical thinking into each phase of product development. For example, during ideation, do an “ethics canvas” exercise to brainstorm potential negative impacts and how to prevent them. During development sprints, include acceptance criteria related to ethics (“Model passes bias tests on X, Y, Z”). Basically, make ethics a design requirement. Founders can institute an internal rule that no product goes out without an ethical risk check.
- Assemble a Diverse, Ethics-Aware Team: Strive for diversity in the team – diversity of gender, race, background, discipline. A diverse team is more likely to foresee a wider range of issues (what one person overlooks, another might catch). Provide training or resources for employees to learn about AI ethics (workshops, courses, reading groups). Encourage open discussion of ethical concerns; team members should feel safe to voice “Is this okay for us to do?” without fear of being labeled not a team player. Some startups even assign an “ethical champion” in each small team – not an expert, just someone tasked to be the conscience and raise questions if something seems off.
- Leverage Tools for Bias and Fairness: Make use of the readily available toolkits to assess and improve fairness. Run bias detection metrics on your data and models as a routine (e.g., check performance across different subgroups). If disparities are found, apply mitigation techniques as discussed (re-balance data, adjust algorithms). Build these checks into your CI/CD pipeline if you have one, so they execute automatically. In essence, treat ethical metrics with the same seriousness as performance metrics. Investors, when evaluating AI startups, can ask “How do you test for and mitigate bias?” – a well-prepared answer here is a green flag indicating the team has a grip on responsible AI practice.
- Implement Privacy and Security by Design: Often overlapping with AI ethics is handling user data responsibly. Startups should ensure they only collect data they truly need (data minimization) and secure it properly. Techniques like anonymization or differential privacy can allow model training without exposing personal info. If your AI uses sensitive data, consider embedding privacy technologies from the get-go (e.g., encryption, federated learning). Not only is this ethical, it avoids violations of data protection laws. Transparency with users about data usage (clear privacy notices) also builds trust.
- Ensure Transparency and Explainability: Provide explanations for your AI’s outputs, either directly to users or at least to client teams using your AI. This could be through an interface or through documentation. Use layperson language for user-facing explanations. Internally, maintain documentation like model cards – it will help new team members, facilitate debugging, and demonstrate accountability. Founders should allocate time in the development schedule for writing this documentation (for example, an engineer finishes a model, then spends a day writing its “README” for ethical use). Investors might request to see such documentation as an indication that the startup is diligence-oriented.
- Test Robustly and Have Human Oversight: Before deployment, test the AI in as many scenarios as feasible – especially edge cases that could lead to harm. Include adversarial testing if relevant (trying to fool the AI or make it behave badly). For high-stakes decisions, keep a human in the loop or on call to monitor outcomes, at least in early stages. For example, if an AI flags resumes, have HR review the flags; or if an AI chatbot is live, have moderators monitor for inappropriate outputs initially. This not only prevents disaster but also helps the AI improve (via feedback). Over time you might automate more, but only with confidence the AI behaves.
- Establish an Ethics/Oversight Committee: As soon as resources allow, form a small ethics committee (even 2-3 people). Their job is to regularly review product plans and features for alignment with the company’s responsible AI principles. They can also be the ones to evaluate any ethics complaints or incidents. For startups too small for a separate group, consider monthly meetings dedicated to ethics review with the core team. Involve advisors or mentors in these meetings if possible for outside perspective.
- Engage Stakeholders and Solicit Feedback: Proactively seek input from those who will use or be affected by your AI. This could mean user testing with a focus on ethical aspects (“Did the AI’s output make you feel treated fairly?”), or consultations with representatives of impacted groups (e.g., if you build an AI for the visually impaired, work with members of that community to ensure it’s respectful and helpful). Show that you’re listening and iterating based on feedback. Similarly, take any user complaints about your AI very seriously – each is an opportunity to fix an issue and demonstrate accountability.
- Plan for Incident Response: Despite best efforts, things can go wrong. Have a plan for how to respond if your AI causes an unintended harm or public controversy. This might include steps like: suspend the system or feature, issue a transparent communication about the issue, investigate and fix the problem, and provide remedy if someone was affected. Being prepared will enable quicker and more responsible action under pressure. From an investor’s viewpoint, a startup that has a risk mitigation and response mindset is seen as more mature and lower risk.
By following these steps, startup founders create a strong foundation for ethical AI development. Meanwhile, investors can champion responsible AI by asking the right questions of startups and providing support (financial or advisory) for implementing these practices. In fact, investors have an important role: they can set expectations that growth must be responsible. Some venture funds now do ethics diligence alongside technical and market diligence. When founders and investors align on the goal of long-term, ethical value creation, the startup is better positioned to build products that are not only innovative but also trustworthy.
To illustrate how these responsible AI practices can be scaled as a company grows, the table below outlines implementation steps at different company stages:
Focus Area | Early Stage (Ideation/MVP) | Growth Stage (Product Market Fit) | Scale-Up Stage (Expansion) |
Ethical Foundations & Culture | Founders set core AI ethics principles; integrate values into mission from day one. Informal team discussions on ethical issues for each feature. | Formalize principles into a written “AI ethics policy.” Onboard new hires on ethical values. Provide basic AI ethics training sessions. Leadership consistently messages the importance of responsible AI. | Company-wide ethics programs and training. Possibly a dedicated Chief Ethics or Responsible AI Officer. Ethical performance metrics (KPIs) introduced (e.g., no high-severity ethics incidents). Ethical culture reinforced through town halls and leadership examples. |
Data Practices & Bias Mitigation | Use diverse data sources even if data is limited. Perform manual checks for obvious biases in datasets. Avoid using features that are ethically sensitive (e.g., race) unless necessary and justified. | Implement data collection standards focusing on representation (cover different user demographics in data). Use bias detection tools on data and model outputs. Introduce a data review step in development pipeline (e.g., verify no sensitive data misuse, check class balances). | Establish a data governance team or process. Regular bias and fairness audits on models in production. Leverage advanced techniques (e.g., bias mitigation algorithms, differential privacy) in data pipelines. Continuously expand and curate datasets to reduce bias as user base grows (feedback loops). |
Model Development & Testing | Choose interpretable or simpler models when feasible to ease transparency. Test model prototypes on edge cases manually. Iterate quickly, but verify no catastrophic failures (simulate worst-case outputs). Incorporate basic fairness or accuracy checks across sample subsets. | Incorporate fairness and robustness metrics into model evaluation (e.g., measure performance by group). Utilize unit tests for AI (testing specific scenarios). Use open-source libraries for explainability to debug models. Conduct an ethics review for any new model before deployment (even if informal). | Formal model validation process in place (bias, robustness, privacy). For high-risk models, involve external auditors or domain experts for independent review. Use scalable test harnesses simulating diverse conditions. Maintain documentation (model cards) for each model version. Possibly implement a model approval committee for go/no-go decisions based on ethical risk assessment. |
Transparency & User Communication | Be honest with users about the AI’s presence (“virtual assistant” labels, etc.). Provide a simple explanation if a user is denied something by the AI (even if manually via support). Create lightweight documentation of the model (intended use, known limitations) for internal use. | Develop user-facing explainability features (e.g., “Why did I get this result?” tooltips or summary reports). Publish model info on website or in white papers for clients (model card elements like performance, data used). Start maintaining datasheets for key datasets. Ensure customer support can explain AI decisions in non-technical terms. | Publicly release transparency reports (could be annual responsible AI report). Comprehensive model cards and datasheets for all major models, shared with clients or regulators upon request. Possibly obtain third-party transparency or ethics certification. In product UI, prominently indicate AI decisions and provide recourse or more detailed explanations on demand. |
Governance & Oversight | Assign an “AI ethics champion” (likely a founder or lead) to evaluate plans from an ethics standpoint. Use ad-hoc advisor input for tough ethical questions. Keep meeting notes on ethical considerations for record. | Form an internal Responsible AI committee or working group with cross-functional members. Schedule periodic reviews of AI projects for ethical compliance. Start tracking key ethical risk metrics (incidents, near-misses). Introduce an internal audit or checklist process for pre-launch. | Establish a formal AI Ethics Board or integrate AI ethics into board governance. Possibly include external ethics advisors. Implement an AI risk management framework (e.g., follow NIST AI RMF processes). Regular audits (internal or external) of AI systems and policies. Align governance with regulatory compliance (e.g., prepare for EU AI Act conformity assessments). |
Regulatory Compliance | Research which regulations/guidelines might apply (e.g., GDPR for data, sectoral rules). Ensure basic legal compliance (data consent, no unlawful discrimination). Build with anticipated rules in mind (for instance, avoid banned practices). | Monitor and prepare for upcoming laws (assign someone to watch AI regulatory news). Engage legal counsel to review AI products for compliance gaps. Implement required measures early (e.g., accessibility standards, record-keeping mechanisms). If operating in regulated domains, begin certification processes. | Dedicated compliance officer or team oversees AI regulatory compliance. Compliance check integrated into product lifecycle (no launch without legal approval for regulated use). Keep detailed technical documentation ready for regulators. Adapt processes to any new law in regions of operation (e.g., ensure all high-risk AI systems meet EU AI Act requirements before entering EU market). |
This staged roadmap shows how a commitment to responsible AI can evolve with the company. At the early stage, it’s about setting the ethical tone and avoiding egregious issues. By the growth stage, the startup establishes formal processes and tools to systematically enforce ethics. And by the scale-up stage, responsible AI is embedded in the organization’s structure, culture, and compliance mechanisms. Importantly, each stage builds on the previous – the habits formed early make it much easier to handle the more rigorous demands later on.
Conclusion: Building AI products responsibly from inception is undoubtedly challenging, especially under the typical constraints that startups face. However, adopting an ethics-by-design mindset is both feasible and beneficial. It helps startups innovate with confidence, knowing that they are considering the broader impact of their technology, and it positions them to meet the rising tide of regulations and public expectations around AI. Investors and founders who collaborate to prioritize responsible AI are effectively future-proofing the business – reducing risk and enhancing the brand’s credibility. As one expert noted, responsible AI should be seen as a “blueprint, not a band-aid”– it’s a foundational design choice, not a reactive fix. By weaving ethical principles into the very DNA of their AI systems, startups can focus on scaling their impact, assured that their growth will be sustainable, societally beneficial, and worthy of users’ trust.
Scholarly Articles & Papers
- Friedman, B., & Hendry, D. (2019).
Value Sensitive Design: Shaping Technology with Moral Imagination.
Cambridge, MA: MIT Press.
(Framework for embedding human values in design.) - Mittelstadt, B. (2019).
Principles alone cannot guarantee ethical AI.
Nature Machine Intelligence, 1(11), 501–507.
(Critical examination of ethical principles in AI.) - Jobin, A., Ienca, M., & Vayena, E. (2019).
The global landscape of AI ethics guidelines.
Nature Machine Intelligence, 1(9), 389–399.
(Analysis of worldwide AI ethics frameworks.) - Holstein, K., Wortman Vaughan, J., Daumé, H., Dudik, M., & Wallach, H. (2019).
Improving Fairness in Machine Learning Systems: What Do Industry Practitioners Need?
Proceedings of the 2019 CHI Conference on Human Factors in Computing Systems. ACM.
(Industry-specific practical insights into fairness in AI.) - Raji, I. D., & Buolamwini, J. (2019).
Actionable Auditing: Investigating the Impact of Publicly Naming Biased Performance Results of Commercial AI Products.
AAAI/ACM Conference on AI, Ethics, and Society. ACM.
(Case study on bias auditing in AI.) - Binns, R. (2018).
Fairness in Machine Learning: Lessons from Political Philosophy.
Proceedings of the Conference on Fairness, Accountability, and Transparency (FAT*). ACM.
(Philosophical basis for fairness metrics in AI.) - Veale, M., & Binns, R. (2017).
Fairer machine learning in the real world: Mitigating discrimination without collecting sensitive data.
Big Data & Society, 4(2).
(Exploring practical fairness techniques and privacy considerations.)
Frameworks & Methodologies
- European Commission’s High-Level Expert Group on AI (2019).
Ethics Guidelines for Trustworthy AI.
Available online.
(Comprehensive framework for implementing ethical AI.) - NIST (National Institute of Standards and Technology) (2023).
AI Risk Management Framework (AI RMF).
Available online.
(Standardized guidelines for integrating ethics and risk management.) - IEEE Global Initiative on Ethics of Autonomous and Intelligent Systems (2019).
Ethically Aligned Design, First Edition.
IEEE.
Available online.
(Influential framework on ethics-by-design.) - Google Research (2019).
Model Cards for Model Reporting.
Available online.
(Standardized transparency and accountability documentation.) - IBM (2022).
AI Fairness 360 Open Source Toolkit (AIF360).
Available online.
(Practical toolkit for bias detection and mitigation.) - Microsoft (2022).
Fairlearn Toolkit.
Available online.
(Open-source framework for fairness analysis.)
Policy Reports & Guidelines
- OECD (2019).
OECD Principles on Artificial Intelligence.
(Internationally recognized ethical principles.) - White House Office of Science and Technology Policy (2022).
Blueprint for an AI Bill of Rights.
(U.S. policy guidance on AI fairness, accountability, and transparency.) - European Union (2024).
EU AI Act – Final Text.
(Detailed legislative approach to AI ethics and compliance.)
Case Studies & Real-world Examples
- Angwin, J., Larson, J., Mattu, S., & Kirchner, L. (2016).
Machine Bias.
ProPublica.
(Influential investigative report on algorithmic bias.) - Amazon (2018).
AI hiring tool scrapped due to gender bias.
(Practical example highlighting real-world implications of bias.) - Microsoft (2020).
Responsible AI Practices.
(Corporate implementation of ethics-by-design.)
Books & Foundational Texts
- Crawford, K. (2021).
Atlas of AI: Power, Politics, and the Planetary Costs of Artificial Intelligence.
Yale University Press.
(Insightful analysis of AI’s societal impacts and ethical considerations.) - O’Neil, C. (2016).
Weapons of Math Destruction: How Big Data Increases Inequality and Threatens Democracy.
Crown Publishing Group.
(Influential text highlighting societal consequences of biased algorithms.)
Implementation Toolkits & Resources
- The Ethical OS Toolkit (2021).
Institute for the Future.
(Toolkit for scenario-planning ethical impacts in tech.) - AI Ethics Canvas (2022).
(Interactive tool for identifying ethical AI considerations.)
AI Horizon Conference
AI entrepreneurs, investors and leaders will gather at the AI Horizon Conference to connect the AI world.
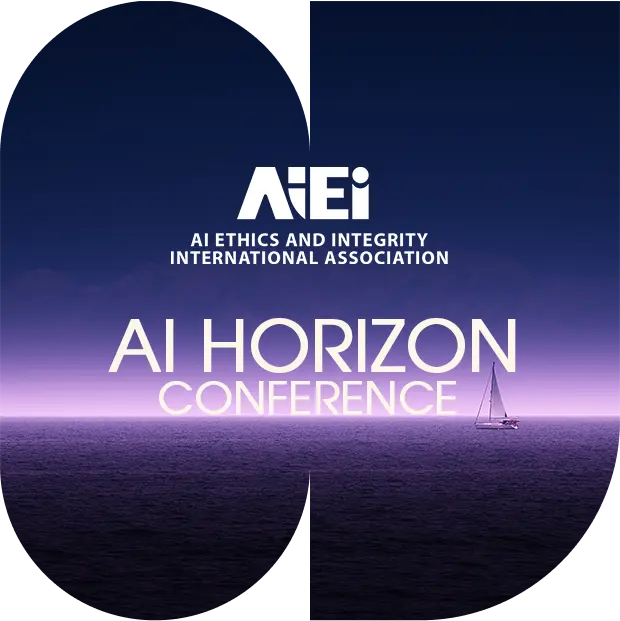